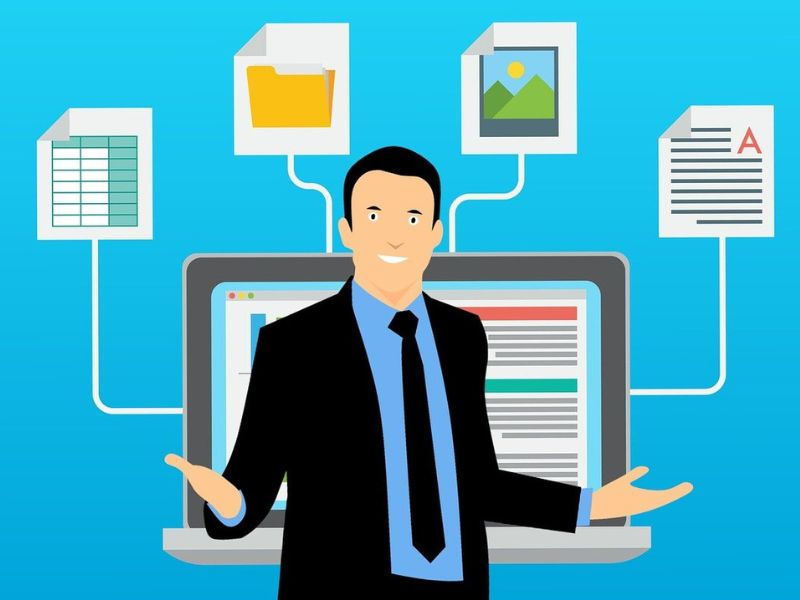
In today's swiftly changing landscape, decision-making has transcended mere reliance on intuition and experience, now incorporating sophisticated data analytics. Recognizing the transformative potential of data to extract insights, facilitate informed decisions, and maintain a competitive edge, businesses, organizations, and individuals are increasingly turning to data-driven approaches.
This comprehensive guide explores the fundamental aspects of data analytics.
Grasping the Core Principles of Data Analytics:
Before delving into the array of data analytics types, establishing a solid understanding is essential. Data analytics involves the systematic examination and interpretation of data to extract valuable insights, formulate conclusions, and facilitate decision-making. Its primary aim is to uncover patterns, trends, and relationships within data to guide strategic actions effectively.
1. Descriptive Analytics:
Descriptive analytics serves as the cornerstone of the data analytics journey, focusing on summarizing historical data to provide a comprehensive understanding of past occurrences. Through data organization and interpretation, descriptive analytics crafts meaningful summaries, reports, and visualizations, offering insights into the prevailing state of affairs.
2. Diagnostic Analytics:
Beyond descriptive analytics, diagnostic analytics delves deeper into historical data to elucidate the underlying causes behind specific events or outcomes. By identifying contributing factors to successes or failures, decision-makers gain clarity on root causes, fostering a culture of learning and continuous improvement.
3. Predictive Analytics:
Embracing a forward-thinking approach, predictive analytics utilizes statistical algorithms and machine learning models to anticipate future trends and outcomes. Analyzing historical data empowers organizations to proactively anticipate potential scenarios, proving particularly beneficial in risk management, resource optimization, and strategic planning endeavors.
4. Prescriptive Analytics:
Building upon predictive analytics, prescriptive analytics recommends actions to optimize outcomes. By evaluating multiple scenarios and predefined objectives, prescriptive analytics guides decision-makers towards data-driven choices aligned with their goals, thereby enhancing efficiency and effectiveness.
5. Real-time Analytics:
In today's dynamic business realm, real-time analytics emerges as a critical capability, involving the processing and interpretation of data as it's generated to offer instantaneous insights. Real-time analytics proves invaluable in industries where timely decision-making is imperative, such as finance, healthcare, and cybersecurity.
6. Text Analytics:
Text analytics, or natural language processing (NLP), focuses on extracting valuable insights from unstructured text data sources. By analyzing textual data from emails, social media posts, and customer reviews, organizations gain insights into sentiment, feedback, and emerging trends, thereby informing decision-making processes.
7. Spatial Analytics:
Spatial analytics scrutinizes geographic or location-based data to uncover spatial patterns, relationships, and trends. Particularly beneficial in urban planning, logistics, and environmental monitoring, spatial analytics offers a holistic understanding of operations by considering geographical context.
8. Social Media Analytics:
Given the prominence of social media, understanding and leveraging online conversations are imperative for businesses and organizations. Social media analytics entails analyzing data from platforms to glean insights into customer behavior, preferences, and trends, thereby informing marketing strategies, brand management, and customer engagement efforts.
9. Cognitive Analytics:
Harnessing artificial intelligence (AI) and machine learning, cognitive analytics simulates human thought processes, offering deeper insights and enhancing decision-making. It surpasses traditional data analysis by comprehending context, learning from experiences, and adapting to changing situations.
Conclusion:
The diverse spectrum of data analytics types plays a pivotal role in shaping decision-making processes across varied industries. From descriptive analytics providing historical perspectives to cognitive analytics emulating human thought processes, each type contributes distinctive insights.
Comments